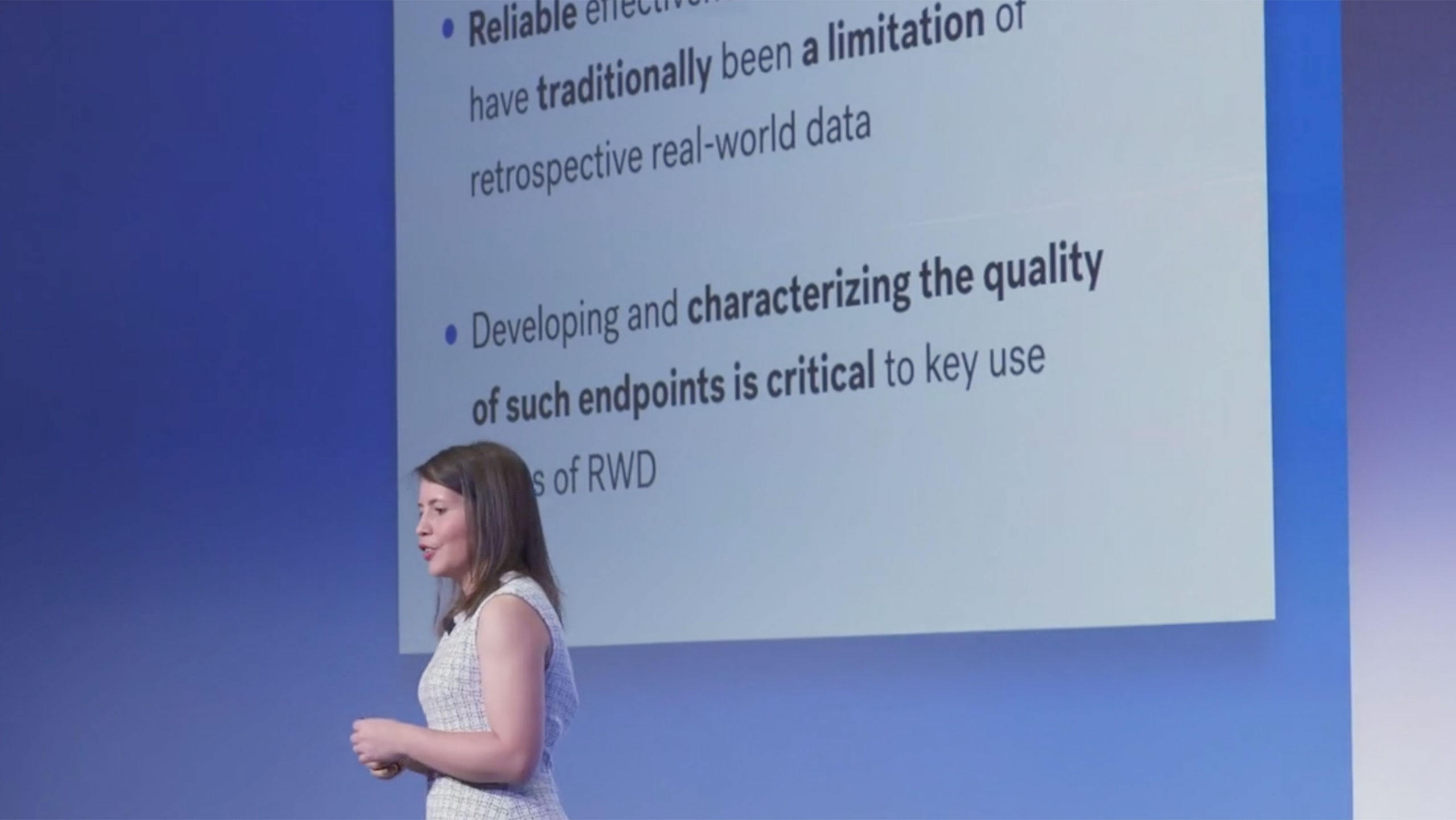
Transcript
Aracelis Torres: My name is Aracelis Torres. I'm a Senior Quantitative Scientist at Flatiron. And as was noted at this morning's keynote, we are trying to demystify some of that process between the source evidence and the creation of our underlying datasets.
So first for the primer, what is it that we mean when we start talking about real-world endpoints in oncology? The first thing to note is that there is no single endpoint that we leverage in analysis and in decision making. This is just a spectrum of the types of endpoints that we are discussing. It is not just mortality, it is also assessing and evaluating tumor based burden. And even understanding, how is the patient feeling or reporting how they are feeling. And as we start thinking about real-world endpoints, we need to discuss them with the backdrop of, how do they compare relative to clinical trial endpoints. What is it that makes them so different? What are some key and important considerations that we should keep in the back of our minds when analyzing them and also in leveraging specific use cases.
One key thing to keep in mind when comparing real-world endpoints with trial endpoints is, in the real world, patients come in to see the physician when they don't feel well. Relative to trials, there's this very specific cadence and expectation that we will likely see them every few weeks, as defined by the protocol. In the real world, we'll also expect that certain patient data elements won't be collected unless the physician really needs it to really inform how they're caring for that particular patient. Well, in the trial, again, it's all defined by specific protocol. You can likely see increased completeness of things like ECoG or lab capture to ensure that that is all available for analysis of trial data. In the real world, there's also variability by clinician interpretation. A physician is looking at the totality of the patient's care, how they're feeling when they step into the office. While trial data is more often than not, evaluated by a centralized investigator and applying the same criteria across all assessments. And in the specific example of progression, it is often determined by a variety of factors by the treating physician. While trial data predominantly leverages RECIST criteria.
I'll spend another minute or two specifically, on RECIST and why it's currently so challenging to understand it in the real world. One portion of it is, there's a technical problem or a technical infrastructure challenge. That images tend to live in a very specific location, separate from the electronic health records. And the time it'll take to think through, how can we meld all of that information together, it's certainly an uphill battle, but certainly not insurmountable. But in addition to there being a technical challenge, there is also completeness issues. More often than not, the imaging reports themselves will not include all the specifics on RECIST to be able to calculate it. And finally there is a longitudinal aspect to it. Radiologists may not be able to access prior images to understand, has the disease changed in any way, with respect to its size, relative to the last time the patient was scanned? So as of today, data for RECIST is not currently routinely captured in real-world care. And certainly, in the meantime, while we figure out how to incorporate more scanning images into our assessments of real-world endpoints, the patients are waiting for some decision making and we need to understand, what are the key components that we can leverage to help inform the effectiveness of therapies in the real world.
So why the need for real-world endpoints? It is traditionally known that clinical trials themselves tend to be fairly slow, pretty expensive, but more importantly, they offer very limited external validity. The patients that make it into trials tend to not be generalizable to all patients that are afflicted with cancer, throughout not only the United States, but across the globe. It is through the real-world datasets that we really understand post-approval. How will this therapy perform in a patient with various comorbid conditions, with liver and renal functions? The type of patient that would not have made it into a clinical trial. And I should note that there's not just a need for real-world endpoints, there is a need specifically for reliable real-world endpoints. It is this need for reliable real-world endpoints that has often been the reason why observational and retrospective studies tend to be seen with some skepticism. How can I be sure that what I am measuring is truly the outcome of interest? How can I understand or get a better grasp of understanding, can I make decisions with it? And what will be critical in the utilization of real-world endpoints is that we characterize the quality of those outcomes and understand the specific use cases that they can be leveraged in.
So in this need and development of real-world endpoints, we need to start evaluating the current spectrum of endpoints that are available, but also look ahead towards the development of new ones. How can we augment current mortality information with external datasets? How can we get a better understanding of treatment-based endpoints? Are there certain treatment settings or disease settings where they are informative and can give us a sense of effectiveness of therapies? How do we continue the path forward to understanding the real-world version of progression instead of RECIST? However, in parallel, still trying to solve the retrospective and prospective issue of images. And then finally, what does the future hold for patient reported outcomes? How can we continue to think about incorporating them at scale and also analyzing them? I should note that all of this should not happen in a vacuum.
We also need to provide analytic guidance with the information of what went into the development of those endpoints. Otherwise, analysis may erroneously include other data points in tandem with those assessments. And without the information of its development, we run the risk of having estimates that are yielding invalid inferences. And we're all here to today because at the end, we care about the patient and we want to make sure that those estimates are correct to inform correct decision making. And keeping all of this in the back of your minds, I'll hand it over to Sean, who will provide the regulatory perspective on real world endpoints, thank you.