In early 2018, Janssen and Flatiron Health began discussions around the development of a new real-world dataset that would allow Janssen to answer critical research questions they had on the treatment patterns and outcomes for patients with Mantle Cell Lymphoma (MCL). What followed was a collaboration between the two organizations who worked to develop a large, clinically-deep and longitudinal real-world dataset leveraging Janssen's scientific expertise and Flatiron's industry-leading capabilities in real-world data.
In this interview, researchers from Janssen, Weill Cornell Medicine and Flatiron Health discuss what went into building this dataset and considerations for using real-world data. In particular, they share the findings from a study which utilized this dataset—a recently accepted Oral Presentation at ASCO 2021.
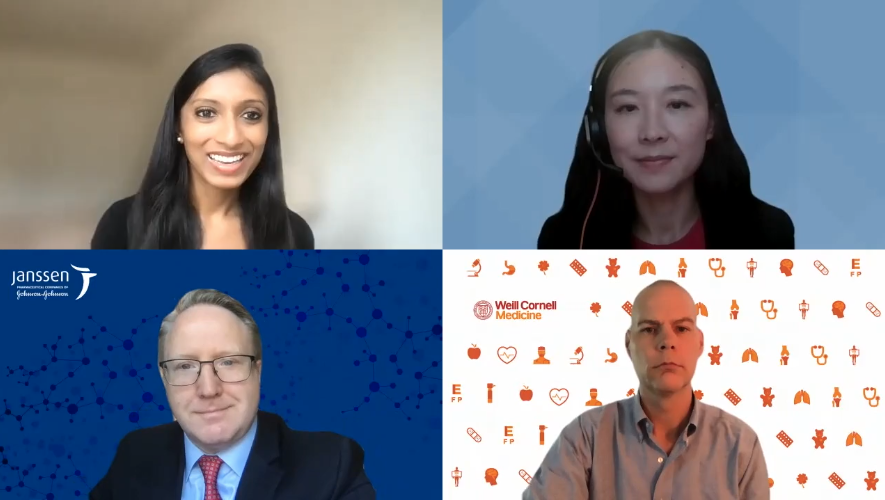
SPEAKERS
-
Peter Martin, M.D, Chief of the Lymphoma Program at Weill Cornell Medicine
-
Mark Wildgust, PhD, Vice President, Global Medical Affairs, Oncology at Janssen
-
Angeline Zhu, PhD, Medical Director, Global Medical Affairs (Hematology) at Janssen
-
Mohona Paul, MS, MBA, Associate Principal, Life Sciences at Flatiron Health
Transcript
Mohona Paul: Hi, everyone. I am so excited to have this group here together for what's going to be a really great and exciting discussion. Looking forward to the several questions to come and just hearing everyone's perspective on what's been a really exciting collaboration opportunity between our organizations here at Flatiron and Janssen. So, to start off, let's begin by just introducing ourselves and providing a little bit of background on our respective roles. I can get us kicked off and then I'll hand off to the rest of the group. So, my name is Mohona, and I am a member of the Life Science Partnerships team here at Flatiron, where I work closely with biopharma partners, such as Janssen, to identify opportunities for collaboration that align with the real-world data and evidence needs of your organizations in a way that can actually leverage the datasets and capabilities at Flatiron. So, I've worked quite closely with Mark and a number of other members of the Janssen team to uncover and execute these opportunities and to build our strategic collaborations together. So, with that, why don't we switch to the Janssen team? Mark, I will hand it off to you.
Mark Wildgust: Mohona, it's really a delight to be with you here today. I'm really excited for this conversation and really excited to have this discussion about the partnership that we have with yourself, but also the partnership that we've had with the academic community, as well, with folks such as Peter who's on the call today from Cornell Medical Center as well.
My name is Mark Wildgust, and I'm the Vice President of Global Medical Affairs here at Janssen, supporting the oncology portfolio of assets, really in helping bring forward new molecules and new medicines to help patients with cancer. I'm a scientist by training. I've been working in oncology research and the pharmaceutical industry for more than 20 years. But at the heart of what I'm doing is I'm really fundamentally trying to help Janssen and really help work with the academic community in bringing new medicines forward to help patients with cancer. That's my passion. And I think parts of the project that we've done with yourself, Mohona, is feeding right into that passion, which is how do we figure out the unmet needs? How do we figure out regimens? And how do we help patients? So, great to be here with you today.
Mohona Paul: Likewise. We're so excited to have you here. Angeline, maybe I'll pass back to you next.
Angeline Zhu:Thanks. I can replicate everything Mark has said, other than my name is Angeline Zhu. I also work with the Janssen Global Medical Affairs Team, with a dedicated focus on Lymphoma. As Mark mentioned, our focus is to bring innovative therapies to patients with Lymphoma, so really excited to have this opportunity to share some of the work we're doing to advance the knowledge we understand about this certain form of rare Lymphoma.
Mohona Paul: Great, wonderful. And then, finally, last but not least, Dr. Martin, I will hand it off to you.
Peter Martin: Thanks, Mohona. It's nice to meet you. I've known Angeline and Mark for a long time. It's nice to see them again. Angeline and I have been working together really closely for a while now on this project in particular, as well as some others. My name is Peter Martin. I'm the Chief of Lymphoma Program at Weill Cornell Medicine in New York City. I'm a physician and proud to represent our organization. We have a pretty large Lymphoma program full of lab and clinical scientists, and we've been working with partners all around the world to help to bring new therapies to patients as well.
Mark Wildgust: And Mohona, I'm going to say something for Peter that maybe he won't say, but I think Peter is one of the leading experts in the Lymphoma community today. He's been a great partner for us. And I think Peter and ourselves share similar aspirations, which is how do we help patients? And we do it from different angles and different vantage points, but the partnership that we can build and put together helps us, I think, collectively achieve that. I hope, Peter, it's okay to say, I think that this is an example of how industry and academia can work together with, really, the same missions, but from a different vantage point as well.
Peter Martin: I agree. Thanks.
Mohona Paul: Absolutely. And Dr. Martin, we're so grateful to have you here. Just to echo all of that before we jump into some of the questions we wanted to cover I think this is an exciting conversation around exactly what you were saying, Mark, in how we keep the patients at the center of what we do in the collaboration and how some great teamwork and opportunities to come together across organizations and across the academic community can ultimately further that goal. So, really looking forward to the discussion to come. So, kicking us off, Mark, I have a question for you. What were the goals or the key scientific questions for this particular research in Mantle Cell Lymphoma? And could you share with us why Janssen was interested in using real-world data in general to answer these particular questions?
Mark Wildgust: Yeah, it's a great question, and I think it's right at the basic heart of everything that we've done here. And Angeline spoke about our passion to really bring forward new medicines to help patients with Lymphomas, and Mantle Cell is one of those rarer Lymphomas where I think there's still more to do. Patients are still dying and we haven't fundamentally brought forward new therapies that really advance care. And so, we wanted to better understand broadly how patients with Mantle Cell were doing in everyday clinical practice. There's one thing to sit here academically and look at the papers and things like that, but we really wanted to take a look and understand that in clinical practice, and particularly for those patients not receiving transplant where we know outcomes are potentially poorer, particularly in those patients who are not eligible for transplant.
And when you look at the question around what a patient's getting today in terms of standard of care, I think Peter would echo this too. Right? What we see is that the standard of care for these transplant ineligible patients is typically chemotherapy induction. Then there's a question about, do I use things like rituximab maintenance? And I think we've seen some pivotal research done over the years that showed that R-CHOP followed by rituximab maintenance appear to improve outcomes for patients. And in many regards, I think the use of maintenance therapy in Mantle Cell was really supported by that particular study.
But what we've also seen over time is that we've seen the clinical community move away from R-CHOP, move towards bendamustine, rituximab, predominantly based upon a study that was conducted in Germany. But the question then remains is, is there really that same benefit of using rituximab maintenance after bendamustine rituxan induction? Does it provide the same benefit? And when you look within the clinical literature itself, unfortunately the data addressed in that question are somewhat limited. I think clinically, it's not unreasonable to think about using rituximab maintenance followed by BR. It seems like a very reasonable thing to do.
But as a company like ourselves, thinking about building regimens and building new approaches to care, when we start to approach regulators or even payers, you can start to look at that data and say, really, the actual robust clinical data supporting that is somewhat limited. And so, we looked around the world to say, "Where could we ask and answer that question? How do patients broadly do? And is there a value, is there a clinical value of using rituximab maintenance?" And it seemed really evident that the US community setting in particular will be a good place to look at that question.
Mohona Paul: Yeah. Absolutely. That all makes a lot of sense, and so, clearly, a really important and impactful question and challenge to solve within the MCL community. And so, just building off of that a little bit, when thinking about the various sources of data that are and were available, what aspects factored into your decision?
Mark Wildgust: Yeah. So, I think we fundamentally wanted to find a data source that could kind of answer that question. The number of trials out there asking that question really didn't do it. And I think there's been some mixed data. Right? I think there was some initial data that suggested that R-maintenance after BR looked like it was improving benefit, but then more recently that data seemed a little bit more indeterminate. So, the question for us really was to try to say, "Okay, where can we find a robust number of patients that we could broadly look at that clinical question?" And it really seemed that the real-world setting would be the most optimal.
And I think over the years here at Janssen, we've partnered with different groups and organizations around the world to look at different questions. Right? One collaboration that we've had for a long time is working with the Karolinska Institutet in Sweden, where you can look at really nice population-based questions. But in this instance, in Sweden, really actually R-maintenance isn't used so much with bendamustine rituximab, so you can't really look at that question.
So, we really came back to focusing on the US community setting, which really led us to engage with yourselves at Flatiron where we know that you have an excellent track record in working with the community, you've got really good coverage. And I think importantly, it would allow us to look at this question, not at one institution, not at one practice, but to really ask this question, you need to look at a large, robust data set with long follow-up. And that really led us back to yourselves, I think, Mohona, at Flatiron because we've had those relationships with you in the past, we know that you have that good coverage within the community, and we know that R-maintenance is being used. So, it was really looking for that organization that would do it, one that we could trust, one that had high quality data, but I think, importantly, had access to the patient data sets that we would need to start to dig into that question.
Mohona Paul: Yeah. Absolutely. I think that's a great summary, and I think exactly hitting the nail on the head in terms of what we think the key value proposition of real-world data is for research questions like this is. So, I think that's actually a really good transition to talking a little bit about how our organizations at Flatiron and Janssen collaborated together to enable the dataset that was used for this research to actually come to fruition, and I think that's a very interesting and compelling story to tell here. And so, I can provide a little bit of context on this from the Flatiron perspective, and then I'd love to hear a little bit more from the Janssen team in terms of how that collaboration played out from the Janssen angle as well.
So, starting off, I'll share some of the history behind the Mantle Cell Lymphoma dataset build. Janssen, I think initially actually came to Flatiron about this in mid to late 2018 or so, expressing interest in MCL real-world data. And at that time, Flatiron did not actually have an MCL data set at scale, and so the data set build began the following year to enable the research question at hand. And at the time of the build, one of the key objectives, I recall, was actually being able to develop a dataset that could be used to address specific research questions through a set of custom projects between Flatiron and Janssen, that was focused on MCL patients specifically. And so, we started to build the dataset by actually leveraging existing data models and abstraction approaches that had previously been developed and used at Flatiron for relevant tumor types. So, for example, Diffuse Large B-Cell Lymphoma and Follicular Lymphoma, but then, of course, addressing the specific nuances that were relevant for the MCL cohort in developing an at-scale dataset for Mantle Cell Lymphoma.
So, throughout this entire process, I remember we worked with Janssen in a really iterative way to make sure that the MCL dataset that we were developing was both fit for purpose, meaning that it supported what Janssen specifically needed from a research objective standpoint, but also that it was generalizable, so meaning the data could actually ultimately be used to support other custom research projects and other research questions that inevitably were going to be of interest further down the line within the same disease space. And so, that whole element of being fit for purpose and generalizable was a really important thing to keep in mind as we built the dataset.
So, just in terms of the general process that we followed to build the MCL dataset, which is typically what we do for building any new dataset, the first thing that we started doing was an initial feasibility phase where we abstracted a limited number of patients in duplicate to understand if we saw any discrepancies in the data that we were collecting and what would be clinically expected for those particular patients, to also understand a bit more around the data availability at hand for the patients in the network, as well as potential limitations that we may be seeing, and then ensure to develop mitigation tactics for these limitations. And then, finally, again, like I said before, to understand any nuances for this particular cohort in the context of real-world data that we would need to account for.
And so, after this initial feasibility phase, as we got to the dataset build itself, we worked with Janssen, again, very iteratively, to read out what we were finding through the abstraction method to understand what the Janssen team's priorities were, again, for the dataset and the things that we were finding through it and the research questions it would enable the team to answer, to align on a final data model and then, finally, to scale it up to reach a desired cohort size. Initially, I know there was a target cohort size that the team had in mind that was desirable in order to enable the specific research goals, and so when we build new datasets, keeping in mind what that target cohort size is and being able to ensure that we can reach that, to make sure that we are supporting the research goals that are in mind is a really critical factor. So, that was something that we wanted to make sure we work through as well.
And then, again, just throughout the entire process, we had touch points with the Janssen team to ensure that we were actually going in the right direction as we were building this dataset, not just from a cohort size standpoint, but also from the data model itself. So, for example, we provided an early readout of the use of oral therapies that were present within the Flatiron network, knowing that that was something that was a specific topic of interest. We also just in general provided monthly status updates on where the data model was going and how it was getting built, and I think as a result of that, it actually meant that we had to make not a ton of changes in bulk and in culmination throughout the process because it was so collaborative and iterative throughout.
So, that's just a little bit on how the process went from our end, but the last thing I'll say, I think it's really important just to reiterate the critical role that Janssen played in sponsoring the build of this dataset. As I mentioned, it was a new tumor type that Flatiron entered into, and serving as really valuable thought partners and collaborators with us throughout this entire build. As sponsors of the build of the dataset, Janssen, of course, was able to provide really key input on key data model decisions that we were making and, of course, get first and early access to the dataset itself once it was ready. But this input was absolutely critical to the Flatiron team throughout the entire process as we built this dataset in a new tumor type, in an area that we knew would really be an area of interest across the market just given some of the trends of approvals that we were seeing at the time. And again, just a great example of partnership and collaboration and something that was really critical to our organization. And so, just wanted to reiterate that importance.
So, hopefully that touches on a little bit of detail on what the process was like from a Flatiron perspective, but Angeline, with that, I would love to turn to you. As a Lead Medical Researcher from the Janssen side, what was your experience working with Flatiron to essentially create this new dataset and help us build it from the ground up? How did you identify what variables would be needed to really answer the research questions that the team had in hand and other research questions in general within the MCL space?
Angeline Zhu:Thanks for the question. I think the first is we really leveraged the experience and the framework you already have. I think that really sets a very strong foundation, a good framework as we move to a new data or disease area or new dataset to be built. Secondly, of course, every disease is different. Right? Mantle cell Lymphoma is different from other types of Lymphoma or the other types of oncology malignancies, so it's important that we define variables that are also unique for Mantle Cell Lymphoma.
I would like to share a few examples of how we tailored. So, in Flatiron, you have the structured data, which is very easily abstracted from the EHR, while you also have the unstructured data which are free text, where you need to abstract the information. I think we really spent time discussing what are the variables in terms of the unstructured data we need to make sure that's tailored towards a Mantle Cell Lymphoma. For example, things like we wanted to be sure that we captured the histology of Mantle Cell Lymphoma because we know that these histologies will have a worse outcome with the current treatment such as a blastoid type of Mantle Cell Lymphoma, so we make sure that information is captured in terms of baseline characteristics.
And additionally, treatment is another big component to it. Stem cell transplantation, as Mark has mentioned, which is commonly used in Mantle Cell Lymphoma, we want to make sure that this information in terms of date, types of transplantation is well captured. Other types of therapies in terms of intravenous or oral therapies used, want to make sure that we have a very clear date of when those are used so we can later really have a closer look at what kind of regimen is being used for different patients at different lines of treatment. I think, overall, when we are thinking about the variable, the gold standard we look to is a clinical trial, how clinical trial baseline characteristics are captured, how clinical trial study endpoints are used. So, we look at what are available in the real-world data set, so we try to mimic what has been captured in that gold standard, but at the same time, recognizing some of the limitations that we might have and try to mitigate in certain ways in the analysis process.
Mohona Paul: Yeah. Absolutely. And then, for this particular study, just honing in on that, which was accepted as an ORAL presentation at ASCO21, what specific methodological considerations did you have as a result of using real-world data specifically? And then just to build on top of that, were there any advantages or disadvantages that you came across by using real-world data to answer this question that you hadn't anticipated going into the analysis and the research?
Angeline Zhu:Yeah. Thanks for that question again. I think, first, we're really glad it was accepted. In fact it was one of the three of our presentations on Mantle Cell Lymphoma at ASCO. I think it's a good recognition from the ASCO scientific community that this is a good quality of data, good quality of analysis that's been done. But of course, when we are looking at the data, I think it's important, it's easily recognizable that a real-world setting in routine practice is different from a clinical trial, which is a more stringent environment. So, that is something we need to take into consideration when we were thinking about the methodological things.
A lot of considerations, actually, around what other information is not readily available in the current real-world data set. I will also provide two examples. One is around the endpoint. In clinical trials, progression-free survival is usually very commonly used as an endpoint to look at the effectiveness of the treatment. So, in this current dataset, we hit a roadblock where we don't have the progression data readily available, so we really looked at endpoints which could be as close to PFS. So, we discussed with external experts and we defined thereal-world time-to-next treatment (TTNT). So, instead of using progression or death as defining the PFS, we defined using start of next line treatment or death in the real-world TTNT definition, which we know that this is in fact emerging as a very important endpoint to look at in the real-world setting. Our physicians are looking at or considering it in the routine practice. So, that is one example where we adapted based on what information is available, but still make sure that whatever information we present is clinically relevant for the community as well.
The second example I have is really around, in a randomized clinical trial, you know a patient's intent is to get a certain treatment. That is what our CTs are for. However, in the real-world, we see many, many different types of treatment. We don't capture the intention. So, that is another thing, when we are thinking about really looking at a certain roles, I think Mark mentioned, we want to look at the maintenance, role of maintenance as an example. We really need to brainstorm or to make sure we adjust for some of the selection biases. Again, this is a great example of working with external experts. We really thought of defining certain cohorts of patients where they all need to get through the first induction period of a treatment so that gives them the equal opportunities to continue. Then it's whether they either choose to get our maintenance or not, then we can have a more objective review of the effectiveness of our maintenance.
So, I think those are two examples where we try to tailor based on what information is available, but still make sure it's clinically relevant. I think the last question you had is about the advantage or disadvantage. I think I talk about disadvantage, I think it's around understanding of the limitations to how to walk around it. The advantage, I think I didn't mention, this cohort is 4,000 plus patients. This is easily the largest Mantle Cell Lymphoma cohort, it includes a lot of data that we can look into to help us better understand the outcome patterns in the US community setting or some of the academic settings as well. So, given this really big data, big data gave us very clear trends that could in the future inform additional things that we need to be answering using this data set, or maybe answer in the clinical trials.
Mohona Paul: Yeah. Absolutely. I think that very, very clearly lays out a lot of the summaries of the challenges and advantages that you get through using real-world data, so thank you so much, Angeline, for that thoughtful answer. Switching gears just a little bit, could you tell us a little bit more about the role that Dr. Martin and other external experts took in the overall efforts to address these scientific questions and the overall research questions for patients with MCL?
Angeline Zhu:I want to use two words. I would only say it's “great partnership” with Dr. Martin, as well as several other external experts from Memorial Sloan Kettering Cancer Center and MD Anderson. They were really, really involved throughout when we were defining the key research questions, to ongoing discussions of how we analyze the data, how do we work around some of the limitations, address some of the limitations, and to presenting the data to ASCO and EHA, and we are having ongoing discussions how to get it published in a peer-reviewed journal at the same time as well.
Of course, at the beginning, we approached them, we told them these are some of the broad areas we are interested to understand about Mantle Cell Lymphoma. Right? We want to understand how the real-world are reflecting the clinical trial findings. We want to see whether real-world data can address some questions not well addressed in trials. And those are things that they are interested in because we all have the common goal. Right? We want to advance the knowledge in Lymphoma care. So, I think that is really... we hit and then that's something we want to continue to discuss and extend our experts' help to continue to refine the detailed research questions. What are some of the key aspects we can look into? How do we define the cohort? What methods would make sense? I would say they are as much involved in the process as Janssen is. Without saying, as Mark mentioned, I see this as a great example of industry and academic coming together, leveraging a great set of data, and trying to advance the knowledge we know and trying to pave ways for some of the future directions as well.
Mohona Paul: Yeah. Absolutely. And I love yourr opening here, great partnership, I think is just such a fantastic way to summarize everything that went into this. And so, with that, Dr. Martin, I would like to switch to you a little bit. And we've heard from Mark and Angeline from Janssen, but as a leading academic researcher in this setting, what did the research actually show from your perspective? And did you identify anything that was either unexpected or surprising in any way?
Peter Martin: Thanks. The research showed really... Well, we had a couple of goals, three goals, really. One was to look at patterns of care. This was, as Mark and Angeline mentioned, a dataset that primarily came from academic... or pardon me, community centers, 80% community centers. We know fairly well how clinical trials read out now, but we've also known for a long time that clinical trials can answer only certain questions and they tend to skew towards certain patient populations, typically more urban, typically younger, wealthier. And so, I think as somebody who treats patients with Mantle Cell Lymphoma, one of my questions always is, can I apply these data from this clinical trial to the person that's sitting in front of me today in the office? And I'm sure a lot of other clinicians ask themselves that question on a daily basis.
So, really, we wanted to look at patterns of care, we also wanted to look at, really, the two questions that Mark mentioned, which were rituximab maintenance, this is something that we know has been associated with improved outcomes, including survival in some contexts in Mantle Cell Lymphoma, and as well, we wanted to look at the role of stem cell transplant. I think the reason why those questions are relevant, really, is because they impact management of patients today, definitely. Also, when I put on my researcher hat, these are questions that, in varying degrees, are being addressed in clinical trials right now: different maintenance strategies, the role of transplant, and some of these questions will come sooner than others. Some may take 10 years to read out. I think what we want to do is we want to be able to be prepared to move forward with the next line of clinical research questions sooner rather than waiting 10 years for the clinical trial to read out, for example.
So, what we found, really three findings. Number one, patterns of care did not necessarily match up with what you might expect based on clinical trials, and there may be a variety of reasons for that. One of them may include the fact, again, that patients outside of clinical trials are simply different than patients who are in clinical trials, patients, again, typically older, less urban. So, there may be patient characteristics that result in treatment selections that are different. Other reasons could include the fact that, oftentimes, the clinical trial or regimens may not be regimens that are amenable to standard community practices. For example, autologous stem cell transplant can't be performed at every center in the United States. Even in New York City where I am not everybody has the social support network to be able to travel long distances.
One of the interesting pieces of data that came from Sweden, which is about as well-supported a social society that you can imagine, was that even marital status was associated with receipt of stem cell transplant. It's clear that there are going to be differences in community practice treatment, academic center practice treatment. Another reason might be that maybe we're not doing a great job of communicating the results of these clinical trials and making it clear why certain treatments might be better than others to our partners in community practice. 80% of patients with Mantle Cell Lymphoma are treated in the community. As an academic doctor, I think one of the things that this taught me was that I maybe have to think a little bit more about how we can develop regimens that are amenable to the community, but also how we can communicate with our partners in the community to ensure that they're offering a standard of care treatment to their patients.
The other two findings, one was related to rituximab maintenance. I think increasingly we are, as Mark mentioned, seeing data that are supportive of rituximab maintenance, following bendamustine rituximab. There was one randomized trial, a small randomized trial, probably under-powered that suggested that there might not be a benefit to rituximab maintenance after BR. Based on that, it has not been offered standardly, but I think now, outside of a randomized controlled clinical trial, I think now we have a pretty large dataset observational and from some clinical trials suggesting that there is a benefit to rituximab maintenance. And that's something that could be impacted upon today. I think that there's a very good chance that it does improve survival of patients, and by doing that today, we have the potential to improve patient outcomes.
And as Angeline mentioned, we're working on publishing those data, including with a validation dataset which I think will support those findings. Lastly, we addressed the issue related to stem cell transplant. I mentioned there are multiple ongoing clinical trials looking at the role of stem cell transplant, but those trials may take a long time to read out, and so it's appropriate to look at observational datasets. In this setting, I think in the eyes of some people, it was surprising, I'll admit that I have always been a little bit skeptical, so I was maybe a little bit less surprised, but we did not find that there was a significant survival benefit in patients receiving stem cell transplant.
A lot of that hinges on how you define who might've been eligible to receive a stem cell transplant, and that's where real-world data can sometimes be a little bit tricky to work with. That's why randomized trials are ultimately the gold standard, as Angeline mentioned. But when we identified the population that we felt that might've been most likely to benefit from the stem cell transplant, then looked at their outcomes with or without stem cell transplant, we didn't find either a time to next treatment or overall survival benefit. And what that means is that, ultimately, we still have to wait for these randomized trials, but I think it's appropriate for us to design trials without stem cell transplant, smaller trials, so that when these big randomized trials read out, we're not a decade behind the timeline, we can start to move ahead with new regimens that have the potential to impact patients and not have these long delays while we're waiting for these trials to read out.
Mohona Paul: Yeah. Absolutely.
Mark Wildgust: And I have a question for Peter. I just want to... So, Peter, one of the things that surprised me, and I'd love to get your reaction to that too, is this idea that potentially two-thirds of patients who were potentially eligible for transplant didn't seem to get it. Right? And so, I thought that was fascinating. What do you think about that? What does that mean?
Peter Martin: I think that is actually consistent with what we've seen with other data sets, interestingly, from the BMT network when you look at the numbers of patients getting stem cell transplants, versus what you would expect based on incidents and prevalence of Mantle Cell Lymphoma, there's a disconnect there. I think many of us have suspected that the number of patients receiving stem cell transplant is fewer than might be eligible. This is, to some degree, an American phenomenon. When you look in Sweden, a higher percentage of patients are receiving stem cell transplant, even with the limitations that I mentioned based on their dataset. So, again, there are a whole bunch of reasons for that. Probably anyone with an imagination can think of different reasons why that might not be the case: social, geographic, a whole bunch of reasons.
Ultimately, if it's true that stem cell transplant is not associated with overall survival outcomes, that's probably not a big deal so long as those patients are then receiving really good other alternative therapies, but that's a key caveat. If we're not doing something that we know works really well, which is stem cell transplant, then we want to make sure that we're using other treatments that we also know work really well.
Mohona Paul: Yeah. Absolutely. And Dr. Martin, you already touched a lot on this, and just, I think, a quick followup that I would just have is, is there anything that we haven't already touched upon that you think are important clinical implications in terms of how the research findings may have influenced, confirmed, or impacted something in your own practice or how you think about MCL treatment options for your patients specifically, and specifically, again, within the role of R-maintenance after BR in elderly transplant ineligible patients? Again, we've talked a decent amount about this, but just in terms of any clinical implications and how you think about this care treatment pattern in a way that we may not have touched upon in depth.
Peter Martin: I don't think that I was so much surprised and so it hasn't necessarily changed my practice. I was already offering them rituximab following bendamustine, a rituximab induction. I've always offered stem cell transplant, but when patients haven't wanted it, I haven't been very upset because I've been confident that we've had other good treatments to offer. But I would say that in the community, people should be considering rituximab maintenance. There are still a number of unknown questions around that, like the duration of rituximab maintenance and what the threshold is for stopping it, so I think there's still... you know Mantle Cell Lymphoma is a really complicated Lymphoma. It's among one of the more complicated Lymphomas. There's still a role for academic and community partnerships there, and we're always available to help out. But I definitely think that most patients still should be managed in the community and we just need to find a way to ensure that they're receiving the same high level of high quality of care that they might be receiving elsewhere.
Mohona Paul: Yeah. And so, just thinking about this, these findings, from the perspective of the broader community, what kind of reaction or interest have you had from the clinical community at large around this data? And how do you think that they might use these insights in clinical practice? And again, asking you to think obviously beyond just your own practice, but what you expect from the clinical community at large.
Peter Martin: We've had a lot of interest from social media and news media who have I think in particular been interested by the differences between what you might expect were the treatment patterns and what were the actual treatment patterns. I think that was probably what has interested people most of all. From my perspective, I think maybe the biggest question that I struggle with is how we can potentially do a better job of building those partnerships. I think moving forward, a lot of the treatment regimens are going to be simpler and can be performed in a community. What we want is when those regimens are moving forward, how can we communicate very quickly so that there's not a two or three year lag in terms of uptake of these newer regimens? I think we want to find ways to do that. Maybe social media is part of that, maybe meetings are part of that. It was one of the questions that really arose during the ASCO discussion, and I don't know if there's a simple answer for that, but anyone that has any ideas, I think we're all ears, for sure.
Mohona Paul: Absolutely. Well, last question here, and this is an open question that I have to all three of you, and so would love to hear any thoughts that anyone has. But if we zoom out a little bit and look back at the study, what advice do you have for other researchers who are interested in using EHR-derived real-world data in their research in the context of what we've talked about? Just all of the learnings, the opportunities that you see. Would love to hear what advice all of you have from this project.
Mark Wildgust: Mohona, I'll go first. I think that setting off with a set of key questions to look at and giving yourself that framework, I think is definitely the key. This idea of looking at these macro questions, patterns of care, who's getting what, what does that long-term outcome look like? Identifying data sources that will help you address that, data sources that you feel like are not going to have some kind of selection bias because of size. So, I think it's looking for data sets that are broad, data sets that have the right type of follow-up, that represent different communities and different practices of care. And as Peter said, when we think about that patient sitting out in Nebraska, that's very different than that patient, frankly, sitting at an academic treatment center. Not to say that the care is not good in Nebraska, it's just different.
And I think that, as somebody at a pharmaceutical company thinking about drug development, many times we're thinking about how do we develop therapies that the regulators think about as the standard of care? But this really reminds me, and I think it really reconfirms that, you've got to look clinically at what people are using, you've got to build on what they're using, and not necessarily just get stuck with, perhaps, what might be seen as a regulatory standard of care. And two things come out of this for me which is, one, rituximab maintenance should be the standard of care for these patients. I think it's absolutely unambiguous coming out of this. I mean, the idea that you would do a study now, a clinical study now not using R-maintenance in the setting, I think, is not right. I think that the data resoundingly showed that.
But I think there's another phenomenon, too, there, and I think it helps us then think about other research. There's this group of patients that don't get transplant that you might think on paper should, and it's almost like there are... we talk about this in the myeloma setting, too. It's almost like this transplant deferred or transplant opting out or transplant didn't receive, even though they could. And I think we have to recognize that, certainly in the United States, that is a real group of patients, and we have to treat them like that, versus this bifurcated transplant eligible, ineligible. I think we have to be more pragmatic about it. And I think, actually, that the data suggests that it really encourages that we need to continue to be that way. Because at the end of the day, as developing new therapies for patients, we're going to develop therapies that actually fit into the standard of care of practice versus doing something that's not interpretable because it's not used. So, I think when you start to think about looking at data sets, you got to think broadly about how you're going to use it and those macro questions.
Angeline Zhu:I will speak a bit about really using the real-world data perspective because we definitely see there's more interest in using real-world data, analyzing real-world data. I think two aspects are one, start planning early. It's easy to come up with the key research questions as people are constantly thinking about it. Looking for the right set of data probably takes time, so I think that is something to keep in mind. So, for example, here, we probably took, I think, half a year discussing it and one whole year to actually having the data built up to 4,000 plus patients. Right? I think the second point is really understanding strength and limitations with real-world data. I think that's extremely important. For us, 4,000 patient data versus 400 real-world patient data, it's different levels of a strength, but at the same time, understanding the limitations help you to look at your methodologies a bit more closely to make sure how to address those, but also be very frank and interpret the data, you're now understanding the strengths and limitations as well.
Peter Martin: From a researcher perspective, the key with working with observational data is how you phrase the question. It seems obvious, but it's actually a really challenging skill and having a very clever statistician, which we were extremely fortunate to have working with us. The team did an amazing job and continued to revise things repeatedly as we narrowed in on our hypotheses questions and how we were going to do them. But from the research perspective, that's key for these large observational datasets as you recognize some of their strengths and weaknesses. From the bigger perspective, I'll paraphrase Dr. Brian Link from University of Iowa who was the discussant of this paper at ASCO, and he said, in order to know where you're going, you really have to know where you've been. And observational datasets, I think, really are key to understand where you've been and where you are.
Mohona Paul: Yeah. I mean, all of this is fantastic advice. I think really valuable. And Dr. Martin, you said it may seem obvious, but it's not necessarily the case. And I think when you look at the grander scheme of how long clinical research has been done, EHR-derived data and using that for this research is still relatively new in that grander picture. And so, I think these insights that all three of you have shared is just tremendously valuable as we think about the opportunity that it can unlock and what some of the key things are that can set you up for success and having really impactful findings and research studies from all of this.
So, with that, I think that brings us to the end of our discussion today, and I really just want to thank all of you for such a phenomenal conversation. I mean, I think it's been a really insightful discussion all around that really helps to show the value that can be unlocked by strong clinical research, great research objectives, and using real-world data to ultimately lead to the critical goal of benefiting patients which, I think as we mentioned at the start, is ultimately what everyone here is really focused on. And so, I really just want to thank all of you here today for such a great conversation, again, and we really do look forward to continuing these types of discussions and the collaboration moving forward. So, thank you. Thank you all so much.
Mark Wildgust: Thank you.
Angeline Zhu:Thank you.